The banking sector has relied heavily on documentation since its inception. Managing large volumes of paper documents was a major challenge in the past. Even today, with online forms, PDFs, and emails, much of the data remains unstructured and complex to utilize.
This unstructured data includes bank statements, tax returns, financial statements, credit reports, contracts, and handwritten notes, making up roughly 80% of the data banks handle. Document management systems (DMS) are still inadequate for processing complex applications, such as mortgage requests, which may involve up to twenty forms. As a result, most financial institutions have a large amount of unstructured data that goes largely unreviewed—only about 3% is appropriately assessed.
This is where Intelligent Document Processing (IDP) steps in. IDP can process unstructured data using AI and Machine Learning, transforming it into a more structured and useful format. In addition to improving data management, IDP helps detect and prevent fraud while reducing processing time.
What is Intelligent Document Processing (IDP)?
Intelligent Document Processing (IDP) is a cutting-edge technology that enables organizations to identify, sort, and analyze information stored in documents of any structure. Manual document handling and analysis can be a slow, error-prone process vulnerable to forgery and other forms of fraud. With IDP, businesses can:
- Identify the data one has to input and take it directly from different written documents (invoices, contracts, IDs, etc.).
- A document's contents or format are compared or checked for inconsistencies or disparities.
- Look for a flag and alert for further investigation.
The Role of Machine Learning in Fraud Prevention
Machine Learning (ML) is another level up from the previous techniques used to prevent fraud. Machine learning algorithms are supervised and unsupervised methods that can be trained to detect and report fraud in real time to look for patterns and suspicious activities in big data. Here's how ML plays a key role: Here's how ML plays a key role:
- Pattern Recognition: They can find behaviours such as high spending or submitting documents that are unlikely to be accepted from historical transactional data.
- Anomaly Detection: By analyzing different documents and using ML to identify them, it is possible to detect changes that fraudsters may have introduced.
- Adaptive Learning: The amount of data input to the system determines the system's level of intelligence. This allows organizations to update their fraud detection methods whenever new threats appear.
Including both OCR technology IDP not only extracts data from documents; the system can recognize the context and structure of the papers by applying NLP. This level of automation speeds up processes but also boosts fraud detection at an earlier stage.
Also Read: Intelligent Data Processing and Automation: The Dynamic Duo Driving Financial Innovation
How IDP and Machine Learning Revolutionize Fraud Prevention
Automated Data Extraction
The primary benefit of IDP is that it can go through various documents and extract relevant information. Machine learning helps IDP extract data and identify information from papers scanned or emailed in any format. It also assists in cutting down the amount of time needed to assess the data. This automation is beneficial since it minimizes the amount of time required to evaluate the data. It also reduces the probability of errors when people assess them, especially when detecting fraudulent activities that use minor alterations.
Enhanced Data Classification
Modern machine-learning approaches allow IDP systems to classify data more effectively than traditional classification algorithms. Given these, it is possible to calculate the probability of such transactions and distinguish between suspicious and non-suspicious transactions more accurately based on patterns and historical data. This improvement in classification capacities directs banks' fraud investigations to concentrate on these risky regions to better develop the dream of fraud prevention management.
Real-Time Fraud Detection
Other applications of IDP can include real-time analysis of transactional data using machine learning models integrated with the platform. Such systems can immediately recognize previously unobserved patterns or actions that can signal fraudulent actions based on constantly processing new data. This real-time capability can greatly prevent fraud before it affects customers or financial institutions.
Improved Accuracy and Reduced False Positives
Many anti-fraud solutions employed in organizations are over-sensitive, thus pointing out numerous transactions for scrutiny when, in a real sense, most of the pointed-out transactions are not necessarily fraudulent. IDP can also be coupled with machine learning, as the latter optimizes the parameters of its algorithms based on the historical data at its disposal. Hence, it also provides a better understanding of true and emerging threats and reduces false alarms, improving resource utilization.
Scalable Solutions
IDP solutions can be scaled with the size of the financial institution and the growth in the financial institution's data. The size of big data and new complex documents also fits well with the preparedness of the machine learning models, which makes the IDP a good solution for the constant changes in fraud prevention in the future. This scalability also has the advantage that the banks can steadily advance and react to new threats or changes without reinventing their current structures to fight fraud.
Use Cases: Preventing Fraud in Every Business and Industry
IDP and ML enhance each other’s capabilities in various industries, such as financial institutions, with less document processing time and high fraud risks. Here are a few examples:
- Banking and Finance: IDP frequently verifies identity documents, while Machine learning determines whether fraudulent loan applications or account takeover.
- Insurance: For claims, Intelligent document processing processes claim forms, and for frauds, ML identifies more cases of fraudulent claims based on historical and behavioral factors.
- Healthcare: IDP deals with confidential patient paperwork, whereas ML identifies possible insurance scams or fake claims of specific treatments.
Conclusion
At nventr, we assist banks and other financial institutions in capturing customer data with higher accuracy to ease document processing and eliminate errors. All integrated with AI and predictive analytics, lenders can make swift decisions while keeping the risks in check.
It is easy to validate information originating from several documents like bank statements and W2 forms with our intelligent APIs that can be adjusted to accord with the loan approval process of a particular institution. This minimizes variations in the portfolio and lowers the likelihood of false positives. They work in harmony with essentially adapted systems and modern banking features, including end-to-end audibility and extremely prompt anomaly detection.
To improve your fraud detection and lending operations, consider nventr’s top solutions by signing up for the free trial.
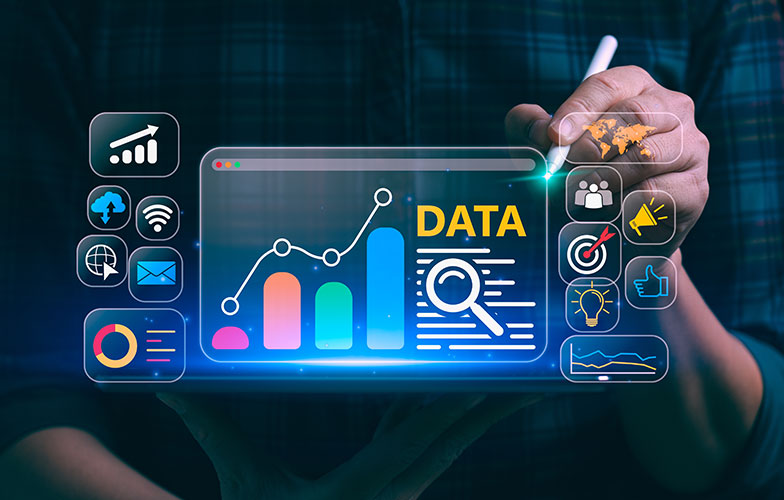
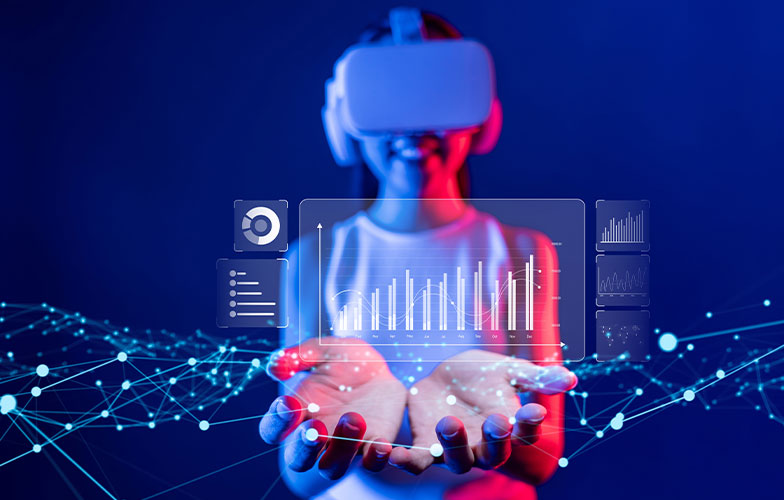
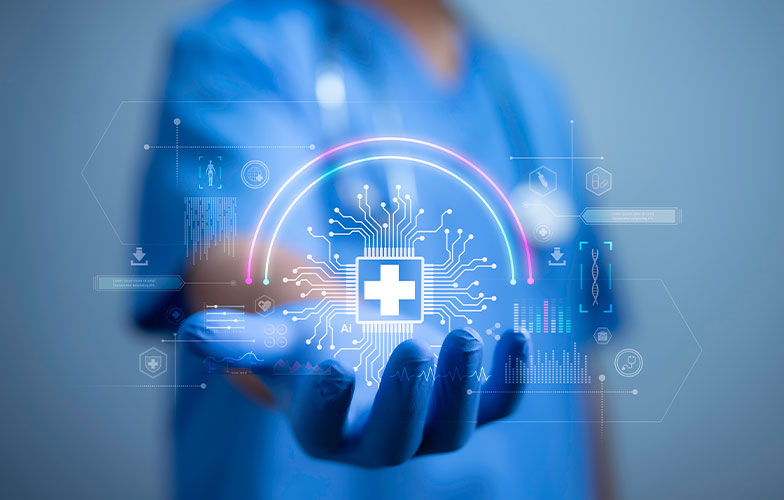
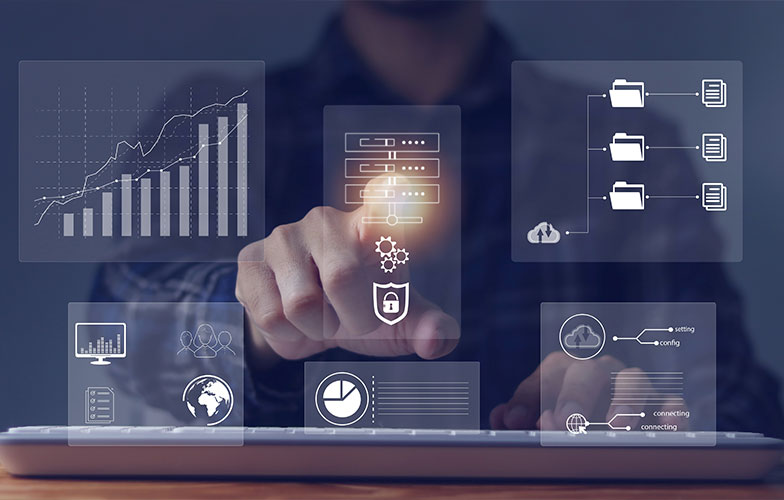
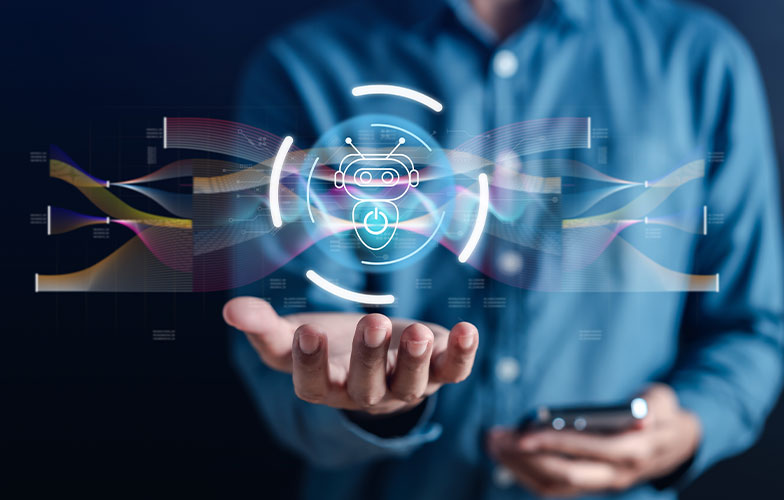