Imagine a world where the tedious job of processing healthcare claims is as seamless as scanning a document and hitting 'send.' While the promise of technology like Optical Character Recognition (OCR) has revolutionized how we digitize paper records, the reality is far more complex. As healthcare providers and payers strive to streamline their operations, they often find themselves entangled in an intricate web of rules-based processing that transforms potential efficiency into overwhelming chaos. The result? A burgeoning backlog of exceptions requiring human intervention is an all-too-familiar scenario for many in the industry.
With the advent of Artificial Intelligence (AI), we can navigate this complex environment in a far more efficient and effective manner. As AI technologies evolve, they streamline claims management in ways once thought impossible. From enhancing decision accuracy to accelerating dispute resolution, AI-driven innovations are reshaping not just how claims are processed but also elevating customer satisfaction for payers and providers alike. The COVID-19 pandemic has only intensified this transformation, pushing the boundaries of healthcare technology.
According to Statista, in 2021, this burgeoning market was valued at around $11 billion, but projections show we’re on course for an astounding leap to $188 billion by 2030—a staggering compound annual growth rate (CAGR) of 37%.
Such rapid expansion highlights not only the increasing reliance on AI technologies but also their vast potential to revolutionize complex processes like claim processing. As we embark on this journey through the innovative realm of neural networks, we'll uncover how these advanced systems can seamlessly navigate and automate intricate tasks associated with diverse data types. Let's explore how artificial intelligence is not only transforming healthcare administration but redefining it as well.
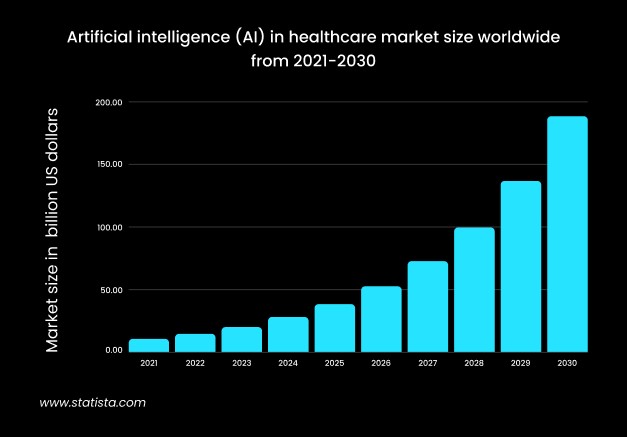
Challenges in Current Systems
Diverse Data Types in Claim Processing
Healthcare claim processing involves diverse data formats, including paper and digital media. Paper documents, such as patient forms, medical records, and billing statements, must first be digitized to facilitate efficient handling. Meanwhile, digital media—comprising emails, PDFs, and scanned images—requires accurate capture and interpretation. Within this landscape, text data is categorized into structured formats (like standard form fields) and unstructured formats (such as doctor's notes or free-text comments), each demanding distinct processing strategies. The challenge increases with unstructured data due to its inherent variability and absence of predefined formats; thus, advanced natural language processing (NLP) techniques are often essential for extracting meaningful insights from this complex information.
Limitations of traditional OCR and Rules-Based Systems
Traditional OCR struggles with diverse healthcare data, causing errors in text capture and workflow. Variations in fonts, handwriting, and layouts hinder accuracy. Additionally, rules-based systems complicate processing due to extensive, predefined rules that must accommodate various data types. As rules accumulate, conflicts and errors become more frequent, making maintenance challenging. This complexity leads to a significant exception queue, requiring manual intervention for claims that don’t fit established rules. Consequently, the claim processing system slows down, increasing reliance on human workers to resolve these exceptions, which creates operational bottlenecks.
High Exception Queues and Operational Inefficiencies
High exception queues in traditional systems reveal inefficiencies, as claims require manual review. This process is time-consuming and error-prone, leading to increased operational costs and delays. The dependence on human intervention undermines automation benefits. Additionally, frequent updates to rulesets for new data types and regulations create a maintenance burden, making these systems rigid and less adaptable to the changing healthcare landscape.
The Role of Neural Networks in Automation
Neural networks, a form of artificial intelligence, revolutionize data processing in healthcare. They mimic the human brain's structure to learn from large datasets and make informed decisions. Unlike traditional systems, they effectively manage complex, diverse data types, making them suitable for automating healthcare claim processing. Their architecture recognizes patterns in structured, semi-structured, and unstructured data. A convolutional neural network (CNN) analyzes images, whereas a recurrent neural network (RNN) analyzes sequential text.
A key advantage is their deep learning capability, which allows automatic feature extraction from raw data. This is particularly useful for unstructured information, reducing manual intervention. Neural networks improve data ingestion by classifying and extracting data from multiple sources, including digitizing paper documents and enhancing OCR accuracy.
It automates the classification and extraction of data from a variety of sources to enhance data ingestion. In healthcare claims, they digitize documents into machine-readable formats with high precision, using advanced OCR to recognize various fonts and layouts. This reduces errors in data capture and improves processing through sophisticated algorithms that interpret and validate information. This minimizes reliance on extensive rules.
Advantages of Neural Network-Based Systems
The integration of neural networks into healthcare claim processing offers numerous advantages over traditional methods. Some are:
- Improved accuracy in data extraction and processing : Neural network-based systems offer high data extraction accuracy, outperforming traditional OCR technologies. Unlike conventional methods, they effectively handle diverse document formats, fonts, and handwriting, reducing errors. Using deep learning models, it is possible to recognize and process information from handwritten notes, scanned images, and unstructured text. Their ability to automatically identify and extract relevant features leads to precise data capture, lowering error rates significantly. This enhanced accuracy ensures correct claim processing and minimizes rework and manual corrections, improving the overall efficiency of the claim processing system.
- Reduction in Human Intervention and Knowledge Workers : It minimizes human involvement in claim processing, unlike traditional methods that rely on complex rules and human workers. Manual intervention in traditional systems is time-consuming and costly, often causing delays and higher operational expenses. In contrast, neural networks automate many tasks, accurately interpreting and processing data to manage more claims without manual review. This leads to faster processing times and lower operational costs, enabling healthcare providers and payers to use resources more efficiently.
- Ability to Learn and Adapt to New Data Types and Processing Rules Over Time : Neural networks excel at continuous learning and adaptation. Their ability to train on historical data allows for ongoing performance improvement, which is crucial in the rapidly changing healthcare sector. With frequent updates in data types, medical codes, and regulations, neural networks leverage machine learning to discern patterns and relationships. This ensures they can adjust to new information. This adaptability helps maintain system accuracy and effectiveness despite healthcare data shifts.
The Future Landscape of Healthcare
Neural networks and deep learning are expected to revolutionize the industry. This will mark the dawn of a new era in medical technology. These advanced AI systems promise to enhance predictive analytics, personalize treatments, and accelerate diagnostic processes, thereby transforming the healthcare landscape.
Empowering predictive analytics
Neural networks analyze large datasets to identify patterns and predict outcomes, particularly in healthcare. They assist in creating predictive analytics tools for forecasting disease outbreaks, patient deterioration, and treatment responses. This technology enables healthcare providers to manage patient care proactively, minimize readmissions, and use resources efficiently.
Personalized Treatments and Precision Medicine
It enables personalized treatments by analyzing genetic, lifestyle, and medical data. This precision medicine approach tailors treatment plans to individual patients, moving away from one-size-fits-all.
Accelerated diagnostic processes
Neural networks enhance diagnostic processes by providing rapid and precise medical data analysis. AI systems, especially convolutional neural networks, effectively examine medical images like X-rays and MRIs to detect diseases such as cancer and pneumonia early. Their efficiency is crucial in areas lacking medical professionals, allowing informed healthcare decision-making. Consequently, patients benefit from timely and accurate diagnoses, regardless of their location.
Enhanced Accessibility and Efficiency
Neural networks enhance healthcare accessibility and efficiency through AI-driven telemedicine platforms. These platforms facilitate remote consultations and monitoring, particularly for underserved populations. By analyzing patient data in real time, they provide critical insights for timely medical interventions. Additionally, it optimizes administrative tasks like claim processing and healthcare record management, lowering operational costs and allowing providers to concentrate on patient care. Automating routine tasks improves healthcare system efficiency, ensuring optimal resource utilization.
Conclusion
The future landscape of healthcare is expected to benefit immensely from neural networks. These technologies are set to revolutionize predictive analytics, enabling healthcare providers to anticipate and respond to patient needs proactively. As we embrace these innovations, companies like nventr are at the forefront, offering solutions that leverage the full potential of AI and neural networks. nventr's Intelligent Data Processing solution exemplifies how scalable neural network-based document classification engines, generalized field extraction, and validation modules can dramatically enhance healthcare claim processing.
Visit our website nventr.ai to learn more.
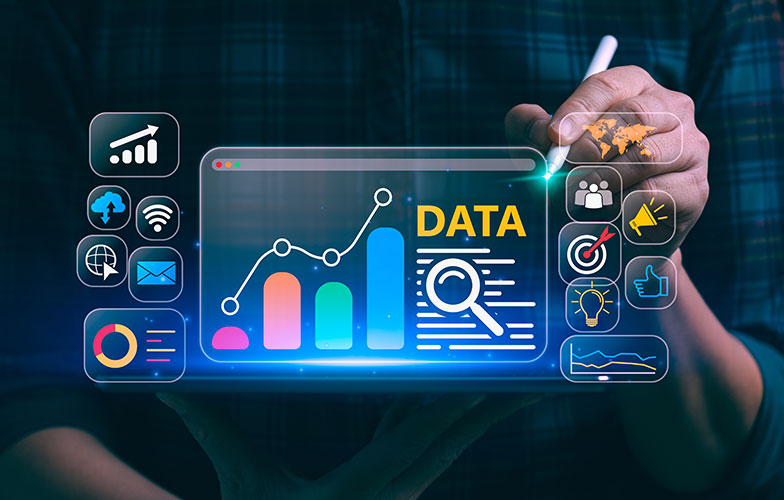
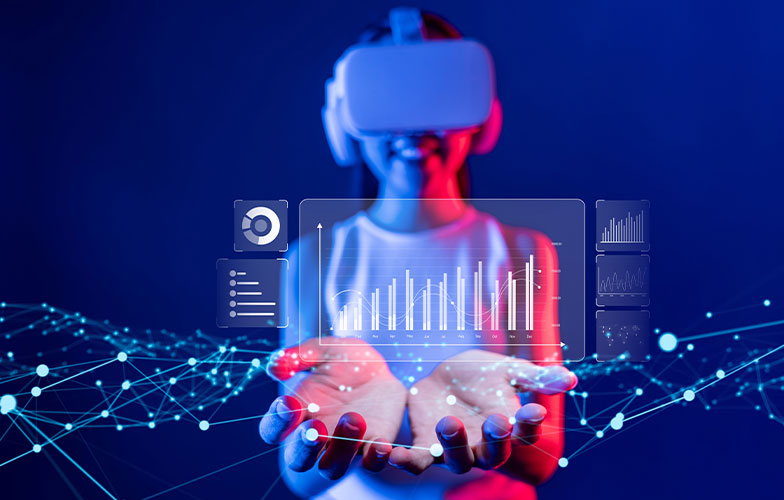
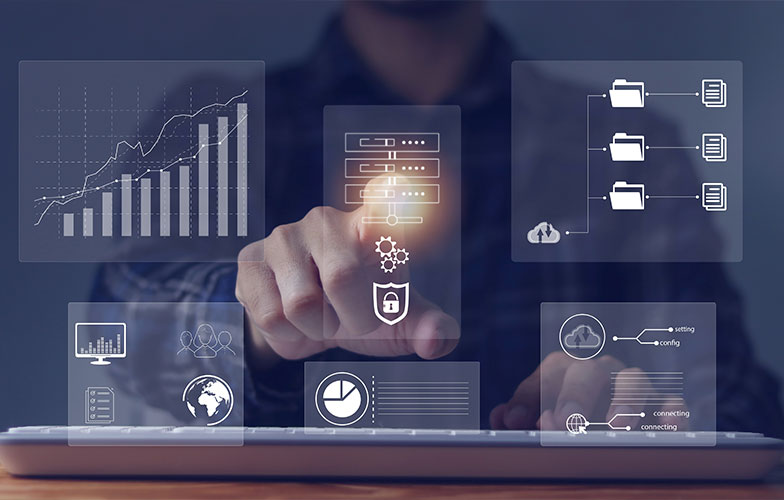